Week 9, Tuesday Discussion
Contents
Week 9, Tuesday Discussion¶
Reminders:
Homework #7 due tonight at 11:59pm
Course evaluations are now open :)
Today:
Pass back old quizzes
Visualizing and interpreting decision trees
Part 1: Data cleaning¶
The dataset included in this project contains grades of Math 2B students. Check to see if there are any missing values. Warning: At first there may not appear to be any missing values.
import pandas as pd
df = pd.read_csv("../data/Math2B_grades.csv")
df.head()
Quiz 1 | Quiz 2 | Midterm 1 | Quiz 3 | Quiz 4 | Midterm 2 | Quiz 5 | Final exam | Webwork | Total | |
---|---|---|---|---|---|---|---|---|---|---|
0 | 7 | 3 | 29 | 5 | 7 | 22 | 6 | 26 | 29.4 | F |
1 | 7 | 10 | 43 | 10 | 10 | 41 | 9 | 68 | 73.0 | B |
2 | 8 | 7 | 32 | 9 | 9 | 42 | 8 | 63 | 67.0 | C |
3 | 10 | 10 | 47 | 10 | 10 | 47 | 9 | 90 | 75.0 | A |
4 | 8 | 8 | 42 | 10 | 9 | 46 | 7 | 73 | 74.0 | B |
df.isna().any().any()
False
Check the dtypes of df
. Does anything appear off?
df.dtypes
Quiz 1 int64
Quiz 2 object
Midterm 1 object
Quiz 3 int64
Quiz 4 object
Midterm 2 int64
Quiz 5 int64
Final exam int64
Webwork float64
Total object
dtype: object
Try running the following code; this is how we can begin to see that there are certain “bad” values in our data.
pd.to_numeric(df["Midterm 1])
pd.to_numeric(df["Midterm 1"])
---------------------------------------------------------------------------
ValueError Traceback (most recent call last)
~/miniconda3/envs/math10s22/lib/python3.7/site-packages/pandas/_libs/lib.pyx in pandas._libs.lib.maybe_convert_numeric()
ValueError: Unable to parse string "ex"
During handling of the above exception, another exception occurred:
ValueError Traceback (most recent call last)
/var/folders/8j/gshrlmtn7dg4qtztj4d4t_w40000gn/T/ipykernel_34199/418289826.py in <module>
----> 1 pd.to_numeric(df["Midterm 1"])
~/miniconda3/envs/math10s22/lib/python3.7/site-packages/pandas/core/tools/numeric.py in to_numeric(arg, errors, downcast)
182 try:
183 values, _ = lib.maybe_convert_numeric(
--> 184 values, set(), coerce_numeric=coerce_numeric
185 )
186 except (ValueError, TypeError):
~/miniconda3/envs/math10s22/lib/python3.7/site-packages/pandas/_libs/lib.pyx in pandas._libs.lib.maybe_convert_numeric()
ValueError: Unable to parse string "ex" at position 167
Discuss: Why haven’t we had to use the na_values
keyword argument recently?
Using na_values
and dropna()
, remove the bad values from our data. Check the dtypes again and think about why it’s not a problem that “Total” is not numeric.
df = pd.read_csv("../data/Math2B_grades.csv",na_values = "ex").dropna()
df.dtypes
Quiz 1 int64
Quiz 2 float64
Midterm 1 float64
Quiz 3 int64
Quiz 4 float64
Midterm 2 int64
Quiz 5 int64
Final exam int64
Webwork float64
Total object
dtype: object
Part 2: Decision tree fitting¶
from sklearn.tree import DecisionTreeClassifier
clf = DecisionTreeClassifier()
Our target in this case will be “Total”. Create a list called cols
that contains all the column names of df
except “Total”. Fit the model with df[cols]
as the input and df["Total"]
as the target.
cols = [c for c in df.columns if c != "Total"]
clf.fit(df[cols],df["Total"])
DecisionTreeClassifier()
Check the accuracy of the model using clf.score(df[cols], df["Total"])
. Why should we be a concerned about this result?
clf.score(df[cols],df["Total"])
1.0
Here are two ways that we can restrict the tree:
Maximum depth
Maximum number of leaf nodes
Instantiate a new classifier clf2
that sets max_depth = 5
and max_leaf_nodes = 10
, then fit it to our input and target. Finally, check the accuracy.
clf2 = DecisionTreeClassifier(max_depth=5, max_leaf_nodes=10)
clf2.fit(df[cols],df["Total"])
DecisionTreeClassifier(max_depth=5, max_leaf_nodes=10)
clf2.score(df[cols], df["Total"])
0.8235294117647058
Part 3: Visualizing and interpeting a decision tree¶
from sklearn import tree
import matplotlib.pyplot as plt
Try running the code below. It corresponds to the overfit tree.
fig = plt.figure(figsize=(200,100))
_ = tree.plot_tree(clf,
feature_names=clf.feature_names_in_,
class_names=clf.classes_,
filled=True)
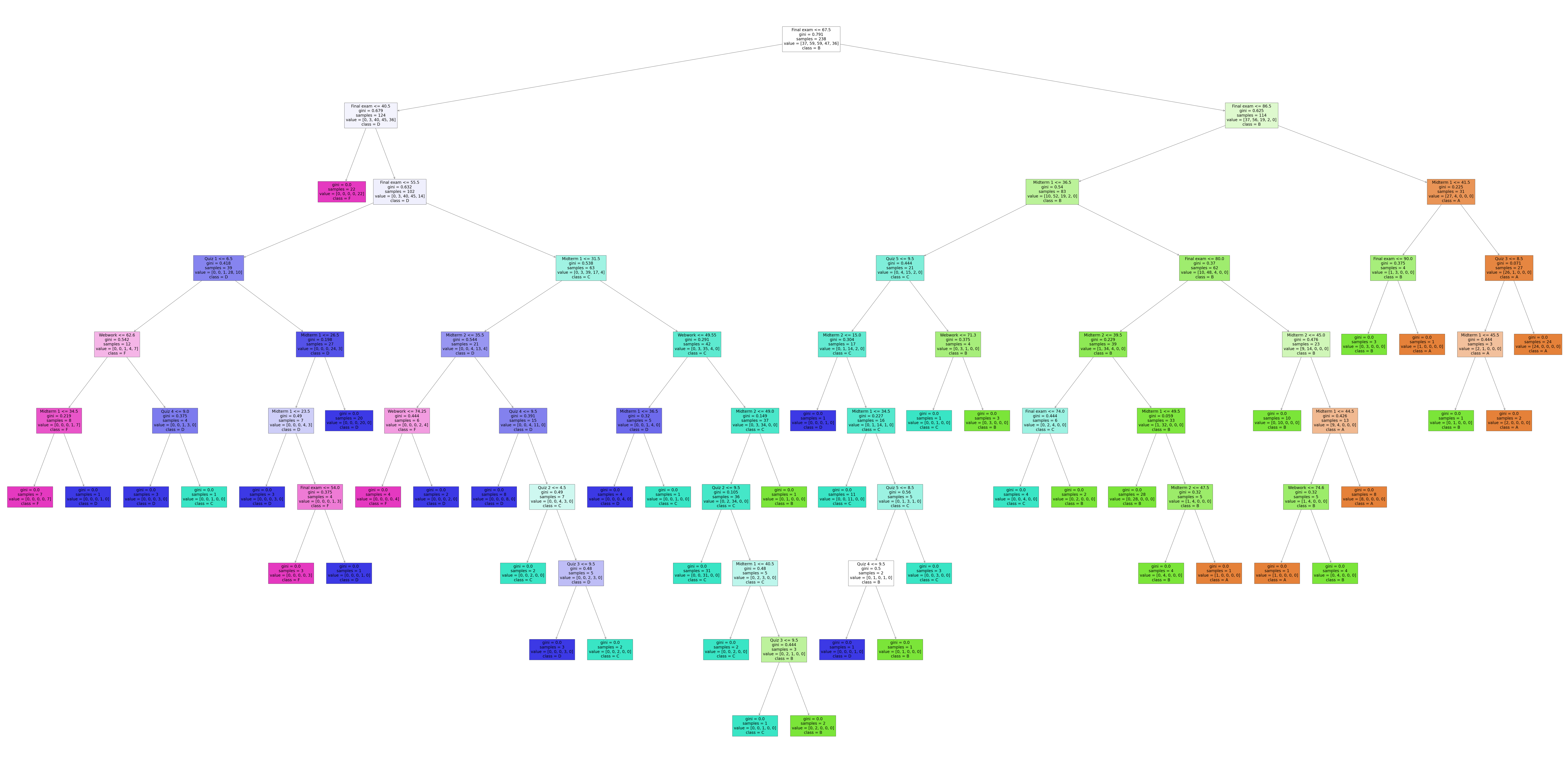
Now try running the code for the restricted tree.
fig = plt.figure(figsize=(200,100))
_ = tree.plot_tree(clf2,
feature_names=clf.feature_names_in_,
class_names=clf.classes_,
filled=True)
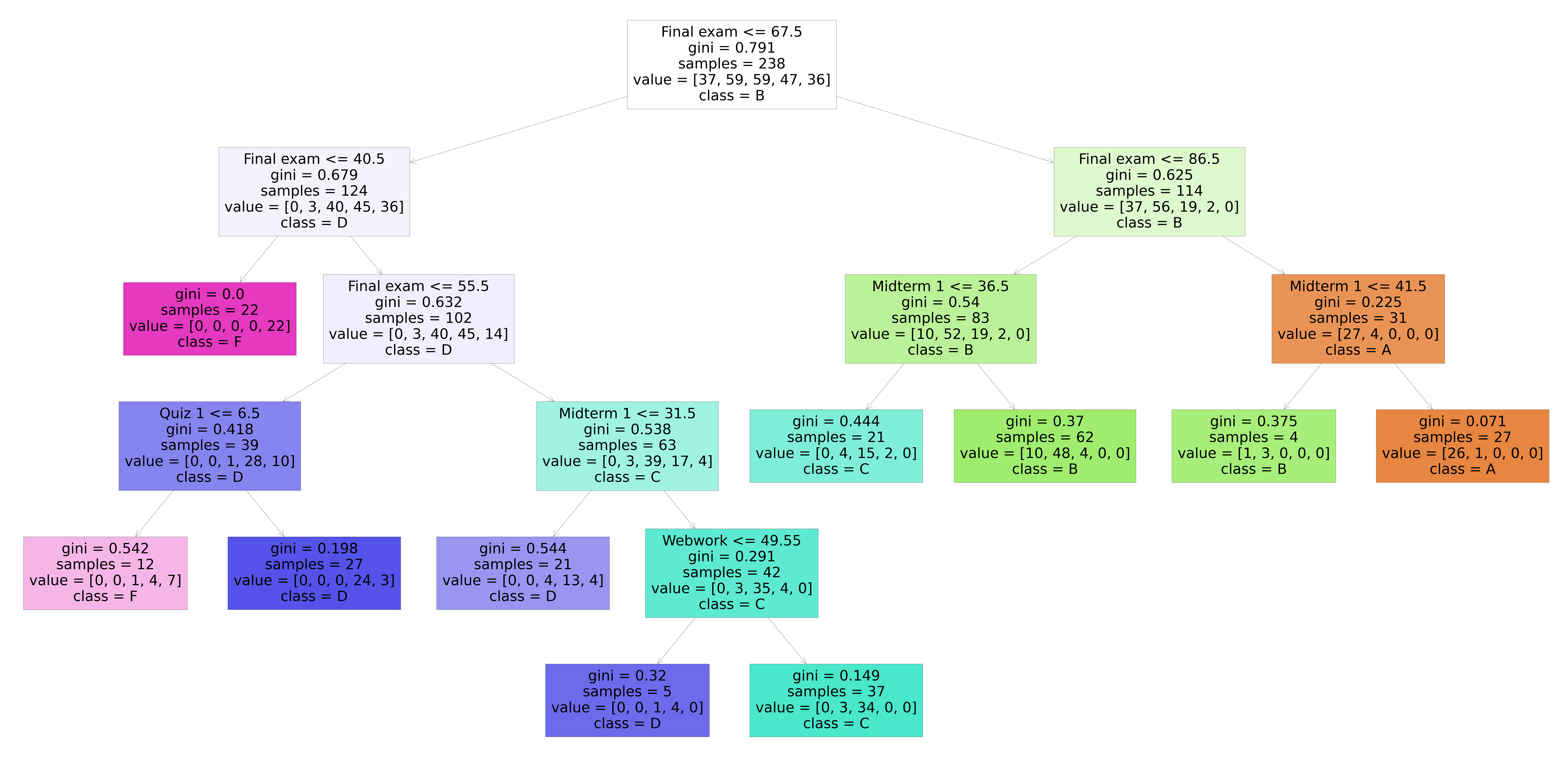
#This is the situation of a student with a midterm of 40 and a final of 80
#We see a probability of about 77%
48/62
0.7741935483870968
student = pd.Series(0,index=cols)
student[["Midterm 1","Final exam"]] = [40,80]
student
Quiz 1 0
Quiz 2 0
Midterm 1 40
Quiz 3 0
Quiz 4 0
Midterm 2 0
Quiz 5 0
Final exam 80
Webwork 0
dtype: int64
clf2.predict_proba([student.to_numpy()])
/Users/christopherdavis/miniconda3/envs/math10s22/lib/python3.7/site-packages/sklearn/base.py:451: UserWarning: X does not have valid feature names, but DecisionTreeClassifier was fitted with feature names
"X does not have valid feature names, but"
array([[0.16129032, 0.77419355, 0.06451613, 0. , 0. ]])
clf2.predict([student.to_numpy()])
/Users/christopherdavis/miniconda3/envs/math10s22/lib/python3.7/site-packages/sklearn/base.py:451: UserWarning: X does not have valid feature names, but DecisionTreeClassifier was fitted with feature names
"X does not have valid feature names, but"
array(['B'], dtype=object)
Don’t worry much about what “gini” means, but everything else should make sense. The top goal for the class should be able to read this kind of tree.
Some questions:
This diagram shows quickly what features are the most important. Which two would you say are the most important? Why do you think Quiz 1 shows up only on the leftmost side, Webwork shows up in the middle, but neither on the right side?
If a student scored 80 on the Final and 40 on the Midterm, what will the classifier
clf2
predict? What would be its predicted probabilities? (Can you replicate this usingclf2.predict_proba
?)Say a tree has depth 3. How many classes at maximum can it predict?
How would you identify a “lucky” or an “unlucky” student from this picture? (A student who got a higher or lower grade than most of their classmates with similar scores.)
How many of the features got used by the classifier?
What if we train a new classifier without the Final Exam among the features. How do things change? Does it get less accurate?
How do things change if we use
DecisionTreeRegressor
instead (say for predicting final exam scores)?